Top AI Tools for Data Analysis in 2024 (Data Revolution)
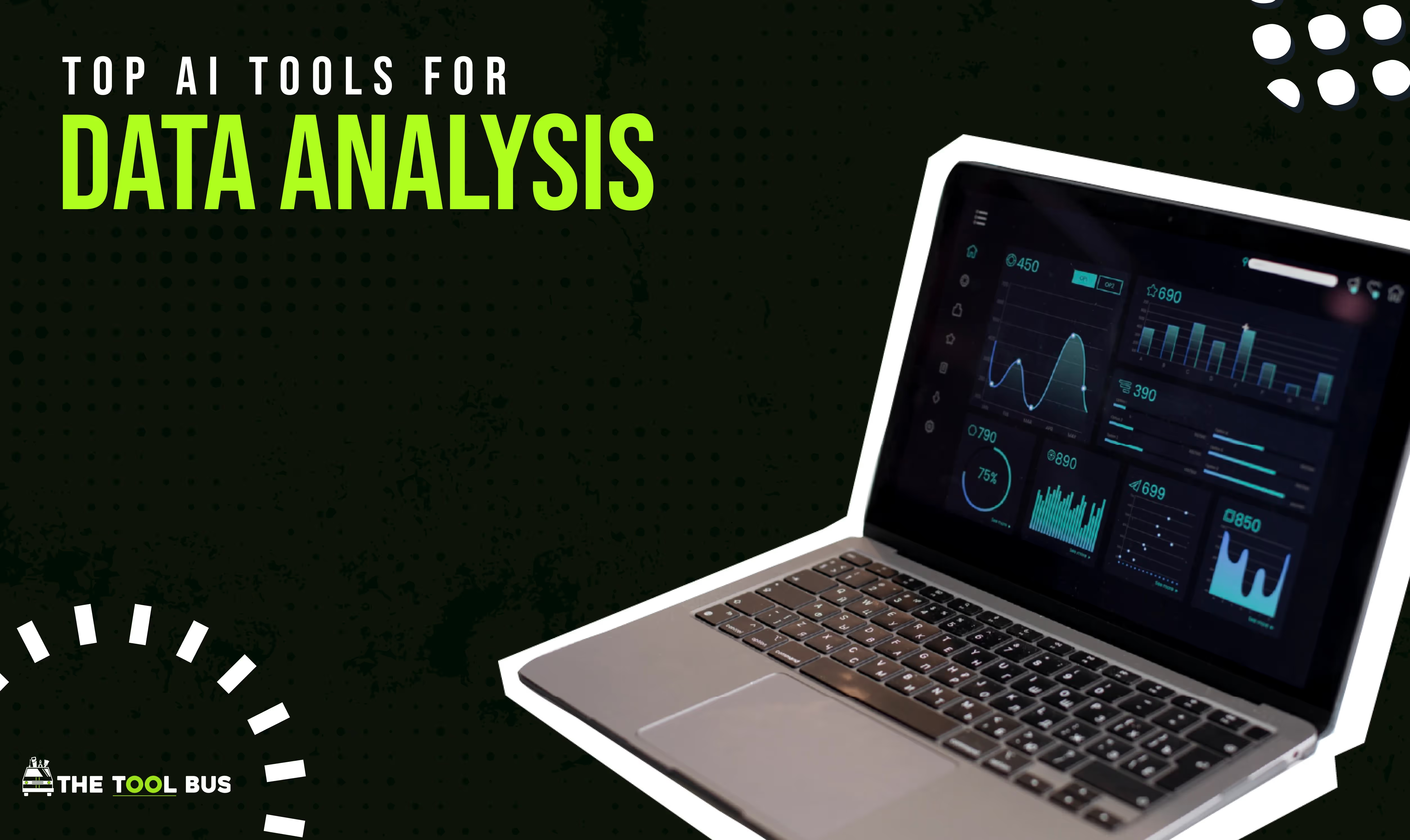
AI tools have become vital in extracting meaningful insights from vast amounts of information. These tools not only simplify complex analysis tasks but also uncover trends and patterns that might be invisible to the human eye. In this article, we explore the best AI tools for data analysis, highlighting their key features, best uses, and weighing their advantages against disadvantages to guide you in selecting the right tool for your data analysis needs.
1. Tableau
Best Use: Data Visualization and Business Intelligence
- Features: Interactive dashboards, data blending, real-time data analysis.
- Advantages: User-friendly interface with powerful visualization capabilities.
- Disadvantages: Can be expensive, and complex data models may require advanced knowledge.
2. TensorFlow
Best Use: Machine Learning and Neural Network Modeling
- Features: Open-source platform for machine learning, extensive libraries.
- Advantages: Highly flexible and powerful for creating complex ML models.
- Disadvantages: Steep learning curve for beginners.
3. IBM Watson
Best Use: Advanced Analytics and NLP
- Features: Natural language processing, predictive analytics, machine learning.
- Advantages: Robust and versatile for various types of data analysis.
- Disadvantages: High cost and complexity may be prohibitive for small businesses.
4. Google Analytics
Best Use: Website and Digital Marketing Analysis
- Features: Traffic analysis, user behavior tracking, conversion metrics.
- Advantages: Comprehensive and free for basic use, ideal for website analysis.
- Disadvantages: Advanced features require time to learn and understand.
5. SAS
Best Use: Statistical Analysis and Business Intelligence
- Features: Advanced analytics, multivariate analysis, business intelligence.
- Advantages: Widely recognized and powerful for in-depth statistical analysis.
- Disadvantages: Expensive and has a steep learning curve.
6. RapidMiner
Best Use: Data Preparation and Machine Learning
- Features: Data preparation, predictive model building, machine learning.
- Advantages: Integrates various functions for the entire data science lifecycle.
- Disadvantages: Full features require a paid subscription.
7. Microsoft Power BI
Best Use: Business Analytics Service
- Features: Data visualization, business intelligence reports, data sharing.
- Advantages: Strong integration with other Microsoft products.
- Disadvantages: Can be less intuitive for users not familiar with Microsoft ecosystem.
8. KNIME
Best Use: Data Pipelining and Reporting
- Features: Open-source data integration, processing, analysis, and exploration.
- Advantages: Flexible and customizable, good for complex data workflows.
- Disadvantages: Might require programming knowledge for advanced tasks.
9. QlikView
Best Use: Business Intelligence and Data Discovery
- Features: Data association, visualization, and guided analytics.
- Advantages: Interactive data discovery and visualization capabilities.
- Disadvantages: Steeper learning curve compared to some competitors.
10. Alteryx
Best Use: Data Blending and Advanced Analytics
- Features: Data blending, data preparation, advanced analytics.
- Advantages: Simplifies complex data blending tasks.
- Disadvantages: Expensive, mainly targeted at enterprise-level users.
Best Picks
- For Comprehensive Business Intelligence: Tableau and Microsoft Power BI offer extensive tools for in-depth business analysis.
- For Machine Learning and Advanced Analytics: TensorFlow and IBM Watson are top choices for their robust and advanced capabilities.
- For Statistical Analysis: SAS stands out for its powerful statistical analysis tools.
Conclusion
AI tools in data analysis are not just about handling large datasets; they represent a shift towards more intelligent, predictive, and insightful analysis practices. These tools enable businesses and researchers to make informed decisions, predict future trends, and gain a competitive edge in their respective fields.
However, the choice of the right tool depends on specific needs, such as the complexity of the data, the required level of analysis, and budget constraints. For instance, TensorFlow is ideal for those delving into machine learning, while Tableau is more suited for creating compelling data visualizations.
In essence, these AI tools are empowering professionals across industries to unlock the full potential of data, leading to smarter strategies and more efficient operations. Whether you are a seasoned data scientist or a business analyst, integrating these AI tools into your data analysis process can significantly elevate the quality of your insights and decision-making.